Data Labeling for Machine Learning | Tagging and Annotation
The big data phenomenon in the 21st century describes the exponential expansion of diverse data, as well as the problems that are connected to it. They include storage, administration, and analysis of any data that may be owned or utilized.
Everything in our modern life from self-driving vehicles to recommendations of the next song in the streaming queue is powered by artificial intelligence. Today, it’s hard to find a company that wouldn’t need to implement AI. Worldwide spending on digital transformation is predicted to reach 1.6 trillion U.S. dollars by the end of the year of 2022, and it’s only predicted to grow.
What is data labeling, then? Labels in machine learning are used to transform raw data (like text, audio files, images, or videos) into a meaningful source of information for an ML model. Relevant and usable labels offer additional context a machine learning model will be able to learn from. Can unlabeled data be used in machine learning? Surprisingly, yes. But you can still encounter the adverse effects of unlabeled data, utilized for training advanced AI-powered systems.
Continue reading to see why data annotation is so fundamental for machine learning!
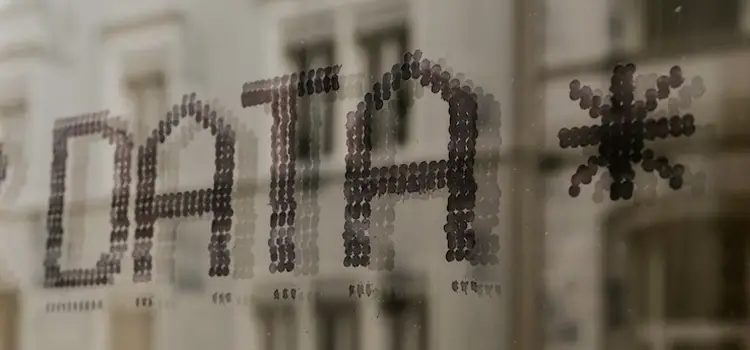
Data Annotation as the Fuel for Machine Learning Models
Between 2022 and 2030, the global market for data collecting and labeling is expected to develop at a compound annual growth rate (CAGR) around 25.1%. The research initiatives in machine learning increasingly depend on data annotation. Smart ML models are able to tackle challenging tasks by enhancing the initial data, particularly through the data labeling process. Businesses are embracing data labeling technology for a variety of purposes, which is causing a boom in the data annotation solutions market.
Even though the process of data labeling is not a rocket science, it’s still a serious matter. A correctly labeled dataset is the foundation for training and testing any machine learning model, which is a crucial phase. Labels, for instance, enable the model to determine if an image depicts a cat or a car, to understand the words spoken in an audio recording, or even determine if a malignant tumor is seen on an x-ray.
For a variety of use cases, such as computer vision and natural language processing, data labeling is a must. It’s crucial to devote the human resources, time, and money required to obtain extremely accurate data labeling. The final quality of any trained model will always depend on how exact was the original data.
Let’s take a look at the main benefits of utilizing data annotation in ML:
- Better accuracy. First, when data is correctly annotated, AI models produce the most exact and efficient outputs. The precision of a machine learning model fluctuates based on how well or how badly the dataset is labeled, or if it is labeled at all. As a consequence, the better the annotation is done, the more error-free is the model;
- Accelerated model training. Compared to unlabeled datasets, models are able to apply valid treatments to the labeled data and provide outcomes that make sense more frequently and quickly;
- Scaled implementation. AI specialists and data scientists can design a variety of datasets of any size for mathematical models thanks to annotated data. It also makes it simpler to produce trustworthy training repositories.
The Main Types of Data Labeling for Machine Learning
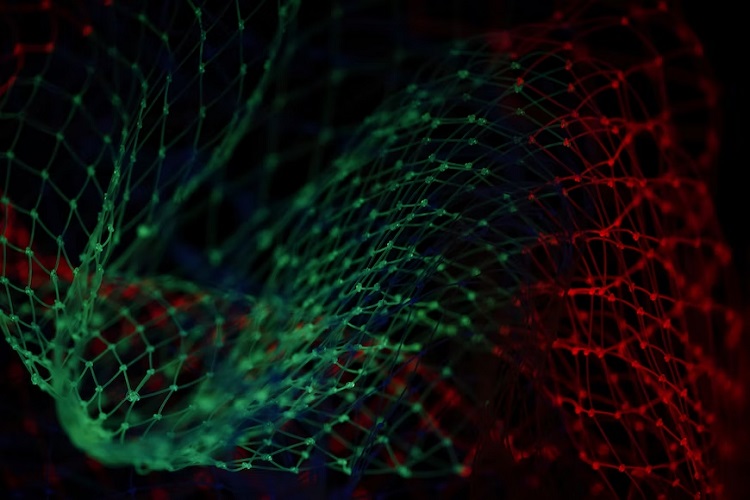
As previously said, data labeling focuses on the function we need a machine-learning algorithm to perform with our data. But as modern businesses deal with huge amounts of data in different forms, each requires a special approach. An audio clip and an image cannot be handled in the same way. So, what are the main modern types of data labeling used in machine learning?
- Computer vision. This area focuses on visual data like images and videos. Computer vision is necessary for processes and programs including facial recognition, self-driving vehicles, and movement detection.
Semantic segmentation, picture classification, 2D boxes, 3D cuboids, polygonal annotation, keypoint annotation, and object tracking are the main types of data annotating in this case.
- Natural Language Processing (NLP). This AI field strives to teach robots how to understand human natural languages. It mainly includes processing of audio and textual data.
Text categorization, sentiment analysis, audio-to-text transcription, named entity identification, and optical character recognition (OCR), which transforms pictures of typed or handwritten communications into machine-readable text, are some examples of data annotation types in NLP.
What Options for Data Labeling Services Exist?
Making the decision of whom to consult during the data annotation process might be difficult. Depending on the issue’s description, the project’s deadlines, and the number of participants, there are many labeling alternatives. The following section lists the options that are used the most.
In-House
In this case, the activity is frequently overseen by data scientists and data engineers that the business has hired. It’s associated with higher quality and control over the annotation process. However, as is typical for in-house labeling, the time required to annotate quickly escalates, resulting in a very sluggish overall process. Not to mention the substantial time and financial resources required to recruit and train a qualified team.
Outsourcing
Here, the data annotation process is outsourced to an expert third-party provider or an individual. It will greatly pay off for smaller companies or short-time projects. This option allows one to focus on the strategy and internal processes, instead of stressing out about all the responsibilities involved with an in-house annotation.
Consider hiring a professional team for secure, accurate, and personalized data labeling for your AI initiative? Check out these annotation services we found for you.
Final Thoughts on a Delicate Matter of Annotation
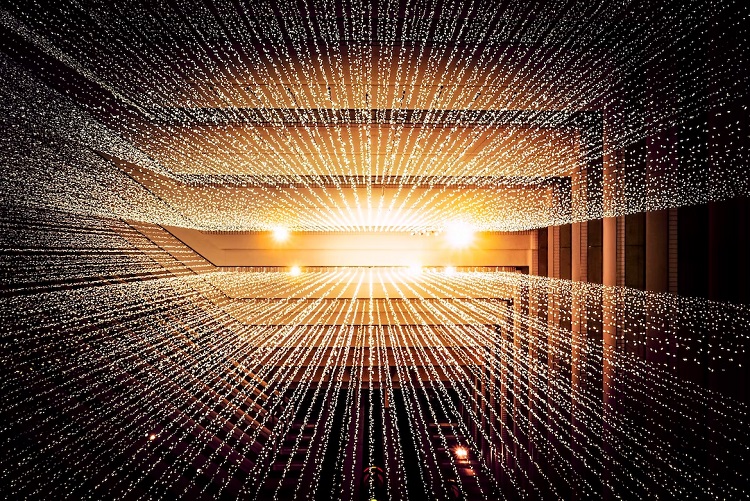
The proper use of data annotation is only possible when a perfect combination of human intelligence, technological experience, and smart technologies is used to generate high-quality training machine learning data.
Data labeling influences whether a high-performing ML model to address challenging matters is developed, or was it just wasted resources on an unsuccessful project. Apart from enhancing efficiency, correctly and thoroughly annotated data enables businesses to boost AI potential and produce machine learning solutions faster in order to satisfy market demands and consumer expectations.
Subscribe to our newsletter
& plug into
the world of technology